Long gone are the days when a biologist could analyze a set of behavioural data by hand. Advancements in technology have put an end to that. Fortunately, teams of statisticians are coming to the rescue of biologists.
Researchers at UBC’s Institute for the Oceans and Fisheries (IOF) and the Department of Statistics have taken a big step closer to understanding the behaviours of northern resident killer whales by improving statistical tools to identify animal behaviours that can’t be observed directly.
The researchers analyzed data from biologgers that were suction-cupped to killer whales. The data loggers recorded video and a host of other variables at fractions of seconds.
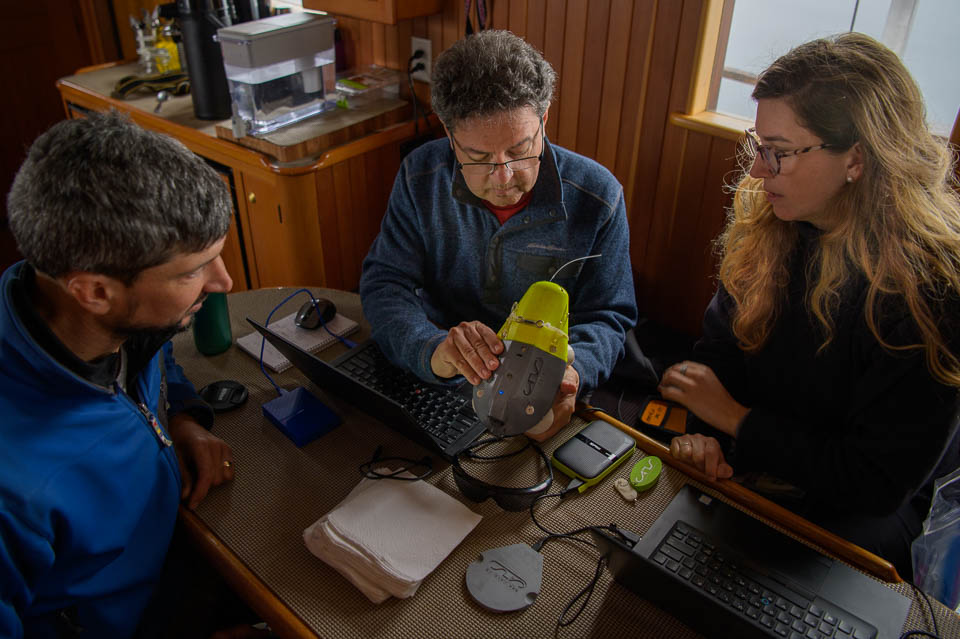
Inspecting a biologger used to collect behavioural and movement data from killer whales.
Two of the variables collected were dive depths and three-dimensional acceleration. Tri-axial acceleration readings (forward/backward, upward/downward, and right/left) are commonly used to infer animal behaviour such as foraging. However, the challenge with so much data is to find the telltale signals among the billions of lines of measurements that reveal when killer whales are searching for fish, capturing fish, travelling and resting—all of which are needed to determine whether killer whales are getting enough to eat.
The research team improved upon a statistical tool called hidden Markov models to unlock the mysteries hidden inside this animal movement dataset.
“Traditional hidden Markov models break down at very fine scales,” said Evan Sidrow, a doctoral student in the Department of Statistics and the study’s lead author. “That’s because there’s structure in the data you can’t capture using the basic type of hidden Markov model.”
The team improved the existing hidden Markov models to better understand northern resident killer whale behaviours. Most notably, their new statistical method revealed killer whales saved energy by gliding when making deep dives, but moved more actively—accelerating faster—when closer to the surface. The improvements made to the statistical analysis of movement data should also allow the researchers to identify pursuits and captures of fish, as well as times spent resting and travelling.
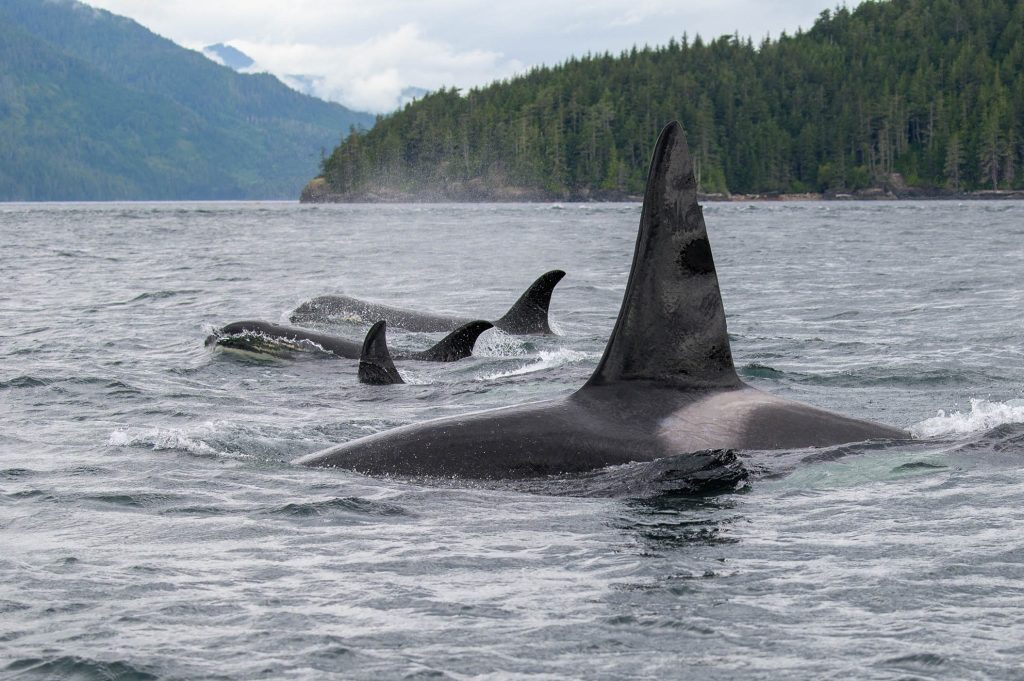
Northern resident killer whales searching for prey near Johnstone Strait, British Columbia.
Future applications of the method extend far beyond killer whales, according to Sidrow.
“It could be applied to pretty much any animal movement data,” he said. “If you’re tagging animals and you want to understand fine-scale behaviours, then this method could be useful—even for things like the flapping of birds’ wings.”
It could even prove useful in areas outside of ecology, such as determining when machines are likely to break by classifying when the parts inside are vibrating abnormally.
The work is one of the first steps on the road to fully understanding why southern resident killer whales are not faring as well as their northern counterparts, according to Dr. Marie Auger-Méthé, co-author of the study and an Assistant Professor in the Department of Statistics and the Institute for the Oceans and Fisheries.
“Using our methods to detect when the animals are catching prey and to model their energy expenditure will be key to understanding the differences between these neighbouring whale populations,” she said.
The next goal is to understand when the whales are capturing prey and applying the models to both northern and southern resident killer whale populations to see how they are behaving differently.
“The paper offers many ‘building block’ solutions that can be used together or independently,” Dr. Auger-Méthé said. “In essence, we are providing a toolbox to researchers using high-frequency movement data, and other similar high-frequency time series.”
“Modelling multi-scale, state-switching functional data with hidden Markov models” was published in The Canadian Journal of Statistics.
Publication
2022 | ||||||||||||||
|
![]() |
![]() |